Trips indicators describe the number of people travelling between two locations in a given period of time, usually hourly or daily.
This is most often expressed as a change relative to a baseline, for example the median number of trips between pairs of locations for the preceding three months.
How are these indicators calculated?
These indicators are calculated from aggregated origin-destination matrices, the pairwise number of trips between every location. These indicators can also be calculated separately for different periods, such as weekdays and weekends or day and night.
Find out more about how we calculate specific trips indicators here.
When interpreting trip indicators, it is important to consider the types of journeys which may be less likely to be captured in CDR data.
This can include shorter journeys which may be completed either without multiple network events occurring or without network events being routed by different towers. This may be a particular concern when comparing mobility between areas with differing densities of cell towers, such as comparing urban and rural areas, or when diagnostic indicators show a change in network activity, as can happen during a crisis.
It is also important to consider the impact of representativeness of CDR data.
If mobile device usage is affected by factors such as age, gender or socioeconomic status journeys, in particular short journeys, by some groups may be less likely to be recorded.
On this page, we present some key trip indicators that can be produced with CDR data:
- Intra-regional travel
- Inter-regional travel
- Connectivity
- Other mobility statistics
Intra-regional travel
We can quantify changes in the amount of movement within an area using intra-regional travel indicators, usually relative to the median for a baseline period.
Intra-regional travel is calculated as the median number of locations visited per subscriber present in the region of interest during a given period of time, most often daily. This requires the aggregates on the count of the number of subscribers in each region and the number of subscribers present in each locality within the regions, during the given time period.
Quantifying the amount of movement within an area has a range of possible applications, but has particularly been used to assess the effectiveness of movement restrictions like those imposed by governments during the COVID-19 pandemic. Similarly, by comparing intra-regional travel after the lifting of restrictions relative to the indicator prior to the pandemic can help assess whether areas are returning to ‘normal’ levels of activity.
Inter-regional travel
Inter-regional travel indicators quantify different aspects of population movements between regions such as the number of people entering and leaving a region; where people entering a region are travelling from; the number of regions people from each region are visiting; and the number of people remaining in a single region.
Depending on the purpose, inter-regional travel indicators can describe the incoming, outgoing flows of subscribers or the net flow (i.e. the difference between incoming and outgoing flows). Usually this is expressed as a percentage change relative to the median flows of subscribers during a baseline period. To calculate these indicators, we need the aggregates of the directed, consecutive origin-destination matrix for each pair of regions and of the count of subscribers for each region, for the given time period.
We can also derive related indicators of inter-regional travel such as:
- Changes to the distribution of distances travelled between regions
- Changes to the dispersion distribution
- Changes to the number of regions visited, per region
- Changes to the number of subscribers visiting a single region, per region.
Connectivity
We can quantify the connectivity between areas from the aggregated pairwise origin-destination matrix. These indicators can describe clusters of interconnected areas and the main routes of travel between different areas.
Clusters of areas are those which are highly interconnected with each other but less connected with areas outside of the cluster. We can identify clusters based on the numbers of trips between different areas.
This has particular relevance to public health in the context of the spread of infectious disease. The presence of cases in one area within a cluster has an elevated risk of spreading to other areas within the cluster. Similarly, clusters isolated from the rest of the country or region may be easier to protect against the spread of an infectious disease.
We can also use the connectivity between areas to identify the main travel routes through a country or region. We can also look for travel routes which pass through hotspots with high crowdedness and mixing.
This again has implications for predicting the spread of infectious disease and could inform public health measures to target restrictions at areas which are important for the spread of a disease to new areas.
Understanding connectivity, and particularly main travel routes, may also provide insights into the demand for transportation which could inform decision-making about the provision of public transport. However, connectivity may also reflect the availability of existing transportation.
Other mobility statistics
We can also analyse subscribers’ trips to generate a broad range of other statistics describing human mobility. For example, we can calculate statistics (e.g. mean, median, maximum, minimum) for the distance travelled by subscribers in a given area or their radius of gyration, a measure of subscribers’ movements relative to a central point.
These indicators can be calculated for different areas to describe changes in mobility. Often, this change is in response to a specific event, such as official warnings about a forecasted disaster or the implementation of government restrictions, for example to slow the spread of a disease.
Discover our two main categories of flow indicators
Trips
TripsHome relocation
Home relocations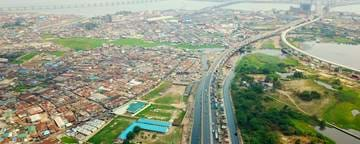